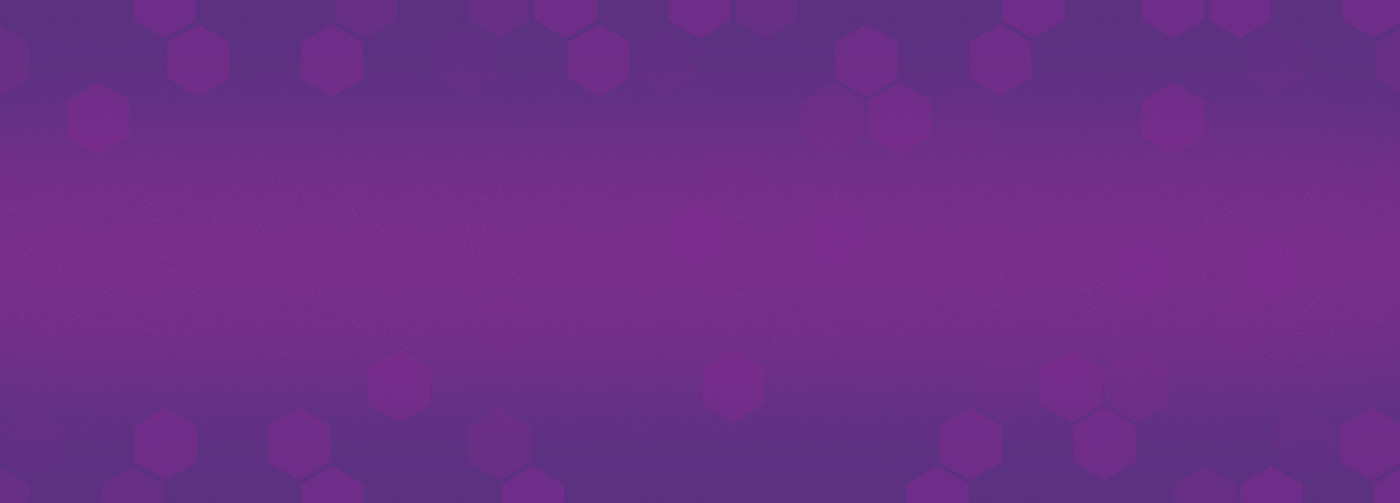
Navigating fluidics challenges can be complex. From persistent issues and system updates to design hurdles or entirely new builds, it takes the right partner to move forward with certainty. We help you achieve your engineering goals.
Full story
Our expertly designed MarvelXACT connection system takes the guesswork out of your process by eliminating the risk of under- and over-tightening, with our patented torque-limiting mechanism. This unique feature provides a haptic “click” feedback when it reaches the optimum torque, assuring a perfect connection every time. Learn More.
Full story
Ever wonder how the vibrant colors on your screen or the perfect shade of paint on your wall are precisely measured and maintained? The secret lies in a critical, yet often unseen, component: tristimulus optical filters. Read our "The Science of Sight: Intro to Tristimulus Filters" to learn more!
Full story
Our BrightLine filter sets have long been an industry ”work horse,” delivering dependable, exceptional performance for a wide range of ever evolving applications and customer needs. We're excited to announce a significant evolution in our BrightLine product offerings, designed to provide unparalleled value and performance: the BrightLine 2.0 and BrightLine Basic Optical Filter Sets.
Full story
IDEX Health & Science’s column hardware products are designed for customers who seek ultimate performance and efficiency. Our column hardware allows you to achieve optimal and repeatable results consistently in your chromatographic separations, and they are ideal for a broad range of Liquid Chromatography (LC) applications, including biopharmaceutical, protein characterization, and proteomics applications.
Full story
Focus on your results and keep your fluidics moving with StabiliX™—our next-generation dual-stage vacuum pump. StabiliX, when integrated with our chambers and control methodology, offers perfect harmony for all analytical applications where dissolved gas and bubbles are problematic. Learn more.
Full story
Prevent issues from bubbling up by integrating an advanced degassing vacuum unit into your instrument with our advanced degassing solution, featuring integrated PCB controls. This system includes a DST vacuum pump and a controller PCB that operates in two distinct modes.
Full story
The current generation of one OEM customer’s instrument could not satisfy the next-generation instrument requirements due to insufficient resolution and color correction over the full FOV. Read more for our solution!
Full story
When you need experts in optical systems and high-volume thin film filters, IDEX Health & Science is there with on-time delivery, guaranteed product performance, responsiveness with issues, and collaborative solutions.
Full story
Deciding on a set of optical filters for a new fluorescence-based product is a complex task that requires careful planning to assure a successful outcome. Complicating factors include the need for fast timeline, reliable production, and budget constraints. IDEX Health & Science has empowered this search process with its line of Semrock® optical filters.
Full story